Amgen's Deep Learning Approach To Vial Inspection
A conversation with Jorge Delgado, Amgen
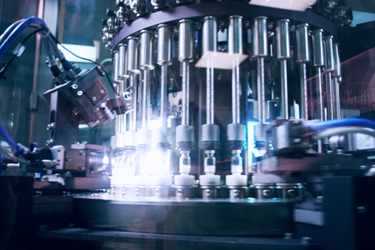
Traditional automatic visual inspection (AVI) has a false rejection challenges.
By some estimates, up to 20%, and sometimes even more, of acceptable products slide down the reject chute because the AVI platform misinterprets a glare as a crack in the glass or bubbles as particle contaminants, says Jorge Delgado. He’s a scientific director of process development at Amgen. He has been exploring how adding deep learning (DL), a type of artificial intelligence, can help bring down the false rejection rate.
He spoke earlier this month at the International Society For Pharmaceutical Engineering (ISPE)’s 2025 ISPE Aseptic Conference along with Amgen process development scientist Jonathan Malave.
We asked him to give us an overview. Here’s what he told us.
Can we start with a brief overview of deep learning? How is it different from the automatic visual inspection standard? How are outcomes different?
DL is a subset of AI that uses neural network-based mathematical models to learn patterns from data, enabling advanced decision-making and pattern recognition. The use of DL in automatic visual inspection (AVI) is not a separate inspection method but an enhancement that complements conventional rule-based algorithms. Many AVI development platforms now integrate DL models alongside traditional tools, leveraging the strengths of both approaches rather than replacing conventional methods.
Traditional AVI tools are highly effective for straightforward inspections, such as detecting color variations in solutions or gross defects. However, DL significantly improves complex inspections, such as differentiating glass particles from bubbles or distinguishing glass glare from cracks — tasks that are challenging for conventional rule-based algorithms.
Our experience shows that when using traditional AVI tools, manufacturers often need to accept higher false reject rates to achieve better defect detection. However, DL enables higher detection rates while simultaneously reducing false rejects, leading to greater inspection efficiency and accuracy.
By integrating DL with conventional AVI, manufacturers can enhance overall inspection performance, achieving more reliable defect detection with fewer false rejects.
How do you handle regulatory concerns about explainability and validation of AI-driven decisions? Talk about the regulatory framework and how it supports DL AVI implementation.
Based on our experience and conversations with industry peers, regulatory uncertainty is one of the most significant barriers to AI adoption in the pharmaceutical industry. Regulatory agencies have expressed support for AI implementation, recognizing its potential to improve patient health and drug quality. To ensure the safe and compliant use of DL in AVI systems, manufacturers must adhere to existing regulations, establishing a foundation for AI integration.
The FDA recently issued draft guidance on AI in drug and biological product manufacturing, incorporating feedback from industry, academia, and patient advocacy groups. This guidance builds on current regulatory expectations and provides specific considerations for AI-driven AVI systems.
A core element of the FDA's framework is its risk-based credibility assessment, which ensures explainability and validation through seven key steps:
- defining the question of interest,
- specifying the context of use (COU),
- assessing AI model risk,
- developing a credibility assessment plan,
- executing the plan,
- documenting the results, and
- determining the adequacy of the AI model for the COU.
This ensures AI models are transparent, validated, and aligned with regulatory expectations.
Following these guidelines enhances AI’s transparency, reliability, and compliance, ensuring DL AVI systems remain explainable, validated, and trustworthy.
Can you talk about implementation? What steps are necessary? Does it require a large investment in computational power?
As with any AVI system, the first step in implementing DL is ensuring the correct vision hardware, mechanical setup, and image quality. DL does not work miracles — it requires a solid foundation of high-quality image data. A dedicated server may be required for data storage, and a graphical processing unit (GPU) is recommended for high-speed applications, particularly in environments with high throughput requirements. Another crucial step is defining the scope of inspection. The DL model must be trained to recognize what is acceptable, what constitutes a defect, and what should be ignored. A diverse data set of physical samples, both defective and defect-free, ensures the model accounts for all possible variability. Images must be captured in the same physical environment as production to ensure consistency.
Once images are acquired, they must be carefully reviewed and labeled. It is important to note that a defective unit may sometimes appear "good" in certain images if the defect is not visible in the field of view. Accurate labeling is critical to ensure the model learns correctly. Next, model hyperparameters are set to control the training process, and the model is trained using the labeled data set. After training, the model is validated to confirm its effectiveness.
A key challenge in DL implementation is the large volume of images required for model training. To address this, image augmentation techniques, including generative adversarial networks (GANs), can be used to artificially expand data sets, reducing the time and cost of development.
The hardware required for DL implementation can be divided into two categories:
Model Training – This step requires significant computational power, particularly for large data sets and complex neural networks. High-performance GPUs or cloud-based AI services can be used to offload this intensive computation.
Runtime Application – Once trained, DL models require much less computational power to operate. The processing requirements are similar to conventional AVI systems, except in high-speed applications, where GPUs can accelerate inference.
What role do human inspectors play after implementing deep learning in AVI — are they still needed?
The role of human inspectors evolves rather than being eliminated, and they are still essential even after implementing DL in AVI. While DL enhances defect detection, it is not suitable for all types of inspections.
DL is less practical for low-volume production environments, where the cost of training and maintaining models may not justify the benefits. Additionally, in-process checks and certain defect classifications still require human adaptability and decision-making.
Beyond traditional inspection tasks, DL introduces new roles for human resources, such as image labeling specialists, model trainers, and AI system maintainers. These professionals ensure model accuracy, address edge cases, and monitor system performance to prevent bias or drift.
Thus, while DL enhances automated inspection capabilities, human expertise remains critical for oversight, decision-making, and continuous model improvement.
About The Expert:
Jorge Delgado leads the Amgen Manufacturing Limited Drug Product Process Development Core Technologies group. The team implements advanced technology innovations through projects such as upgrading drug product filling lines, automatic visual inspection lines, and packaging lines. He has a master’s degree in manufacturing engineering and a bachelor’s degree in industrial engineering from the Polytechnic University of Puerto Rico in San Juan.